Microfluidic CODES is a novel system of orthogonal microfluidic channel detection that leverages code division multiple access (CDMA), a spread-spectrum telecommunication technique also used by cellular networks to distinguish user signals. In CDMA, signals are transmitted simultaneously and within the same frequency band, while multiplexing is achieved by modulating the information in each channel with a unique digital spreading code. This code helps track the number of cells that have passed through the channel as well as the distinctive properties of each cell, for example, allowing the detection of abnormal and potentially cancerous cells.
Since this Georgia Tech system combines waveforms from the distributed sensors into a single data stream that requires computational processing to recover information, it also uses a two-stage deep learning algorithm for signal processing and to analyze the data it produces. This algorithm demonstrates greater than 90% pattern recognition accuracy and increase signal processing speed to allow for real-time particle analysis. Based on convolutional neural networks, this machine learning analysis technology improves the scalability, performance, and overall utility of Microfluidic CODES-based systems.
- Scalable: Set-up allows for the integration of multiple microfluidic devices to increase throughput volume.
- Versatile: Machine learning analysis can be applied to other microfluidic devices integrated with the same Coulter sensor network.
- Pragmatic: This innovation improves the capabilities of lab-on-a-chip systems to provide an affordable solution for low-resource settings.
- Comprehensive: The single electrical waveform that is produced contains information from all sensors distributed over the microfluidic chip.
- Environmental monitoring
- Biotechnology
- Bioanalytical instrumentation
- Health care
- Diagnostics
- Blood testing
The Coulter sensor is a reliable method for characterizing particles in a microfluidic channel. It uses resistive pulse sensing technology to detect the electrical conduction change as a particle passes through a micro-constriction. Georgia Tech’s Microfluidic CODES leverages CDMA in an orthogonal pattern to minimize cross-correlation and maximize auto-correlation, distinguishing particles even as they overlap. Using machine learning to supplement the robust Microfluidic CODES system, this innovation optimizes particle assessment and allows for the easy gathering, analyzing, and storing of data from the device.
To see more technologies by Dr. Sarioglu and his team, please click here.
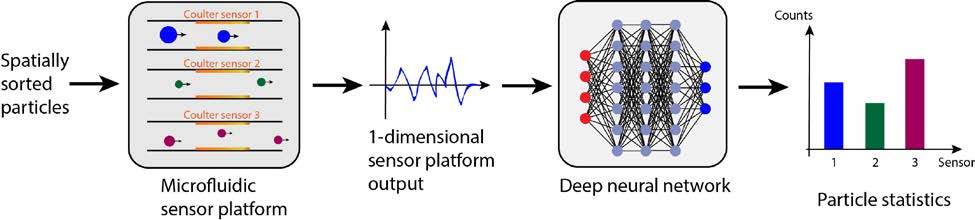
An overview of the system
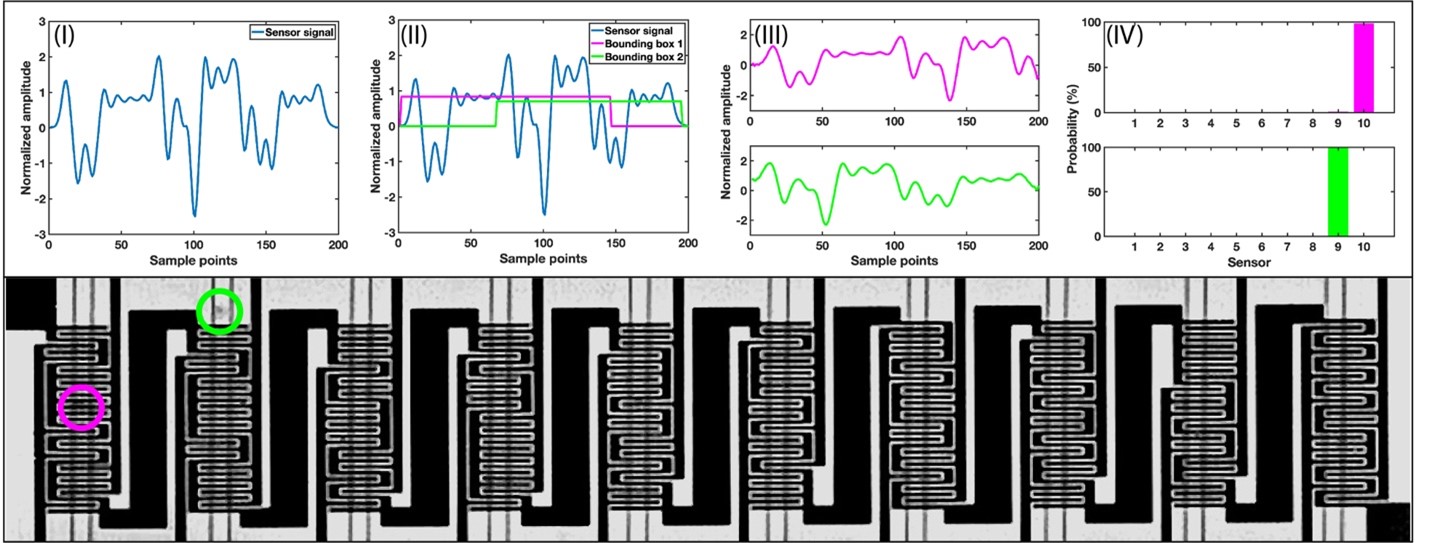
ConvNet decoding process of a sensor waveform with simultaneously-recorded high-speed camera image.