This artificial intelligence (AI)-assisted millimeter-wave (mm-wave) Doherty power amplifier (PA) architecture achieves robust adaptive operation over antenna voltage standing wave ratio (VSWR). A built-in machine-learning AI core uses online function estimation and reinforcement-learning (RL) algorithms for dynamic, Doherty-specific performance optimization. This Georgia Tech innovation is the first hardware platform realized with self-adaptive control algorithms.
The AI core automatically adjusts both the main and auxiliary PA settings for optimal performance and characterizes the system based only on the observed input/output power as data sequences for large-signal gain linearization.
The control algorithms provide the PA self-reconfigurability, permitting robust adaptive operation over environmental changes. Multiple RL frameworks have been incorporated in the control algorithms including multi-armed bandit (MAB), continuum-armed bandit (CAB), contextual-bandit (CB), and actor-critic with experience replay (AC). The control algorithms based on the latter three frameworks leverage prior information about the Doherty PA’s characteristics to improve learning efficiency. A specific algorithm can be selected based on the trade-off between control efficiency and computational/memory complexity.
For proof of concept, researchers demonstrated performance improvement using a 3-bit mixed-signal Doherty PA (MSDPA) as a hardware platform. Over 2:1 antenna VSWR variations, simulations showed that the AI core significantly improves Doherty PA’s linearity and efficiency.
- AI-assisted: Provides the PA self-reconfigurability and robust adaptive operation over environmental changes
- High performance: Features low latency and fast response capabilities required for many fifth-generation (5G) applications
- Improved learning efficiency: Incorporates into control algorithms multiple RL frameworks, including CAB, multi-armed bandit, contextual bandit, and actor-critic with experience replay
- Wireless systems
- Complex mobile applications
- 5G multiple-input multiple-output (MIMO) systems
As mm-wave applications become prevalent in commercial and defense markets, advanced transmitter and PA architectures are needed, especially for communications applications that use high peak-to-average power ratio waveforms. The Doherty PA architecture is an attractive option due to its high efficiency at power back-off and large modulation bandwidth. However, the PA architecture is highly sensitive to the auxiliary PA onset power and the load modulation between the main and auxiliary paths. In addition, the load modulation relationship often varies drastically over antenna VSWR mismatches, necessitating complicated calibrations.
Recent developments in AI and online machine learning have inspired new approaches for designing adaptive systems, as AI algorithms learn a system’s characteristics based on the data collected during the system’s operation.
Georgia Tech’s AI-assisted PA architecture utilizes a built-in machine-learning core to achieve adaptive operations over VSWR mismatch and optimize performance.
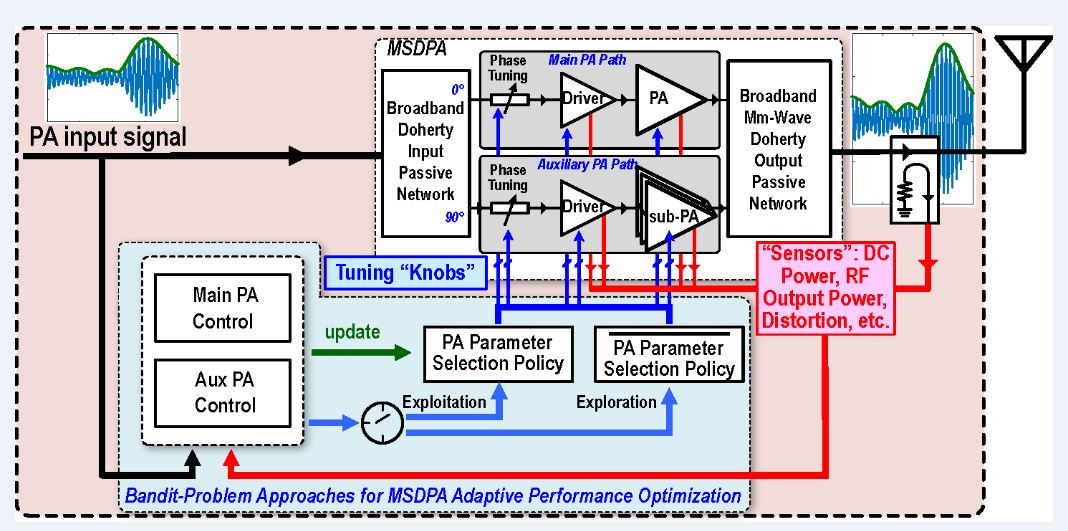
Conceptual diagram of the AI-assisted mm-wave Doherty PA. The mixed-signal Doherty PA (MSDPA) contains main and auxiliary PA paths. Both inputs and the sensed outputs are sent to the control unit, which runs RL-based algorithms to achieve extended linear gain region while maintaining high PA efficiency.