Inventors at Georgia Tech have developed a data-driven analysis of causality in video, which enables effective video content processing in an unsupervised fashion, with emphasize on temporal interaction vs. user interactions. The method includes two steps:
1) Point-process representation of video by ’visual words’ occurring in a subset of frames.
2) A spectral representation that captures statistical relationships between such visual words using a cross-spectral density function.
Space-time visual words representing the motion video are sequenced by recognizing low-level visual events (i.e. ‘words’), similar to object categorization. The temporal causal analysis further segments such visual words into independent, semantically meaningful groups. This method demonstrates a qualitative segmentation performance along with quantitative improvements in retrieval and categorization of social events. It is able to aggregate information across a long time interval, sustaining continual analysis as well as capturing quasi-periodic or repetitive motions. As a result, various ‘video events’ can benefit from improved retrieval and classification performance.
- Enables characterizing the temporal structure of video events in an unsupervised manner, - a key to video analysis
- Reduces the need for training data
- Improves performance in retrieving and classifying social events from a video
- Computationally and memory efficient
- Video segmentation
- Higher-level vision tasks such as activity recognition, tracking, content-based retrieval, and visual enhancement
Causality (cause-effect) is analysis of motion sequences in order to establish meaningful relationships between the "visual events", - i.e. associated temporal dependencies, such as ones observed in the game of soccer. Nonparametric formulation of Granger causality can be used to identify patterns of interaction between repeating events, and partition them into independent causal sets.
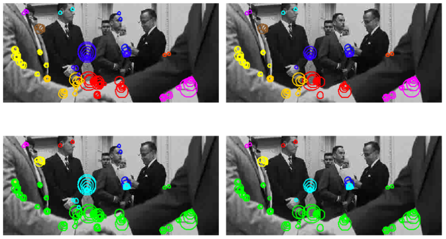