Inventors from the Georgia Tech Research Institute have created a domain tolerant, yet domain aware, approach to data modelling and decomposition with a method called Configurable Hyper-referenced Associative Object Schema (CHAOS). This invention is intended for use in the Multi-domain Analysis and Deep Knowledge Association Toolkit (MADKAT), a GTRI Internal Research and Development (IRAD) project for analytic discovery in massive multi-source and multi-domain data sets. CHAOS is a lightweight decomposition schema for unstructured and structured data to provide extract, transform, and load (ETL) capabilities needed for MADKAT. Due to the human-centered nature of the MADKAT project, an overarching goal of CHAOS is to be comprehensible to the target audience and provide continual self-documentation of purpose. This means that underlying data representations should always explicitly state their identity and purpose while also serving their function of maintaining complex knowledge relationships. This allows the decomposition of data to follow logically from the CHAOS structures and provides traceability through the resultant representation.
- Efficient application of tools and algorithms
- Simplifies the extract, load, and transform phase of data processing
- Business-business development, conflict of interest, market research, market segmentation
- Computing - data warehousing, search engine, analytic platform
- Legal - patent research, conflict of interest, compliance audit
- Health Informatics - health database aggregation
- Defense/Law Enforcement - investigation, sensor data fusion
Technical Analysts across various industries are challenged by massive datasets, including multi-source and multi-domain data. Two particularly vexing problems are the confluence of (A) Data Veracity, i.e., highly sparse, misaligned, noisy, and uncertain data, and (B) Data Variety, i.e., unstructured and variable format heterogeneous data. Our proposed solution is a human-in-the-loop, semi-automated (also known as mixed-initiative in cognitive science parlance) system of tools, processes, and knowledge management for analyst augmentation and data fusion. This construct is a collaborative framework between a human and a machine capable of interactive data enrichment, whereby initial autonomous machine data curation enables the domain expert to consider highly voluminous and variable data interactively in order to associate, de-duplicate, and otherwise simplify semantic, casual, and functional relationships. This work support emphasis enables rapid execution of tasks central to domain agnostic analytics while also exposing important, but often hidden, information critical to knowledge discovery.
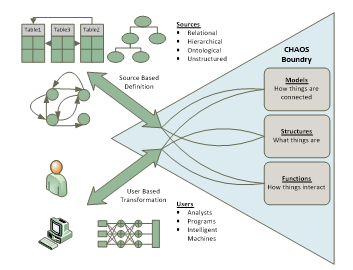
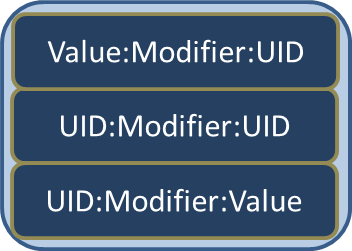
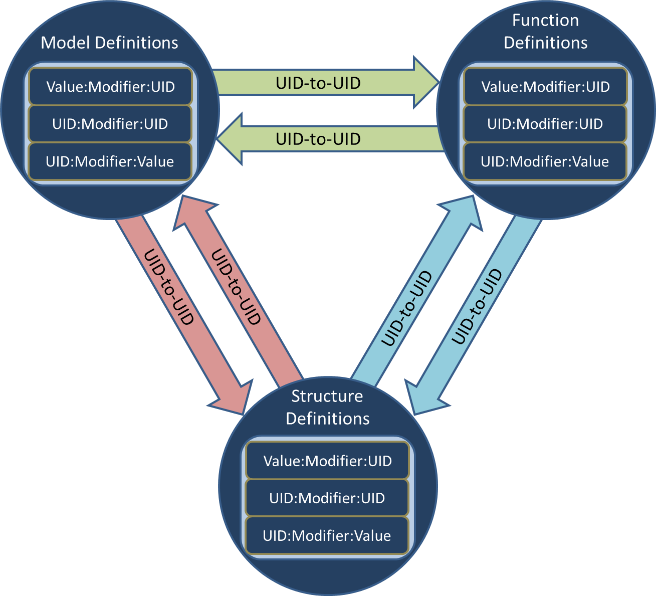
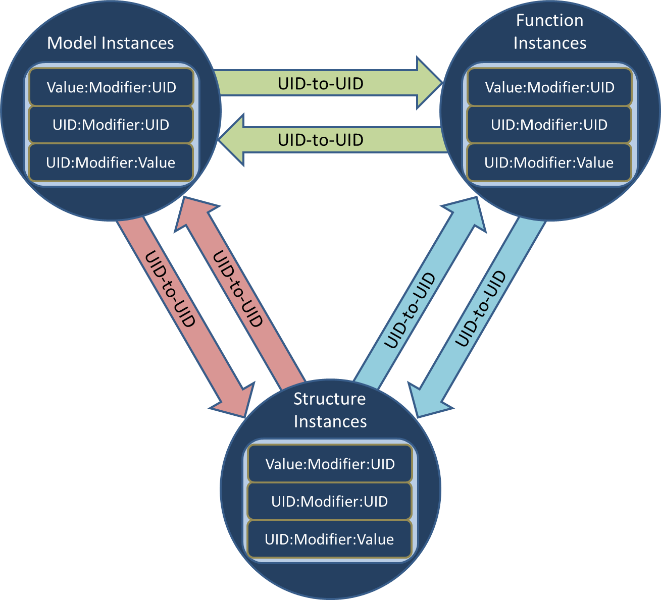
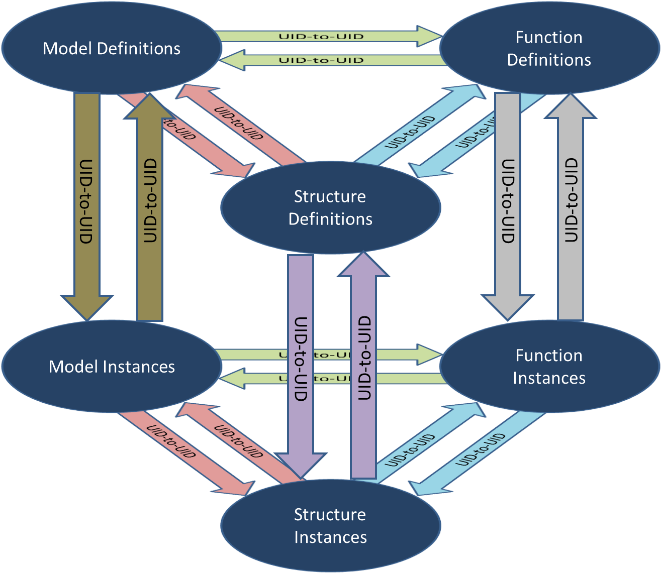